Village Data Analytics
Two billion people are cut off from any power grid. They live with makeshift generators or without electricity at all. This is a massive challenge, especially for health care in times of a global pandemic: 600 million people are served by health centers that do not have the electricity to charge electronic medical devices, connect to the Internet for communication and information, or run refrigeration for storing medicines and vaccines. Improving the situation and providing affordable and sustainable access to energy is not only Village Data Analytics's (VIDA) mission, but also one of the United Nations’ Sustainable Development Goals.
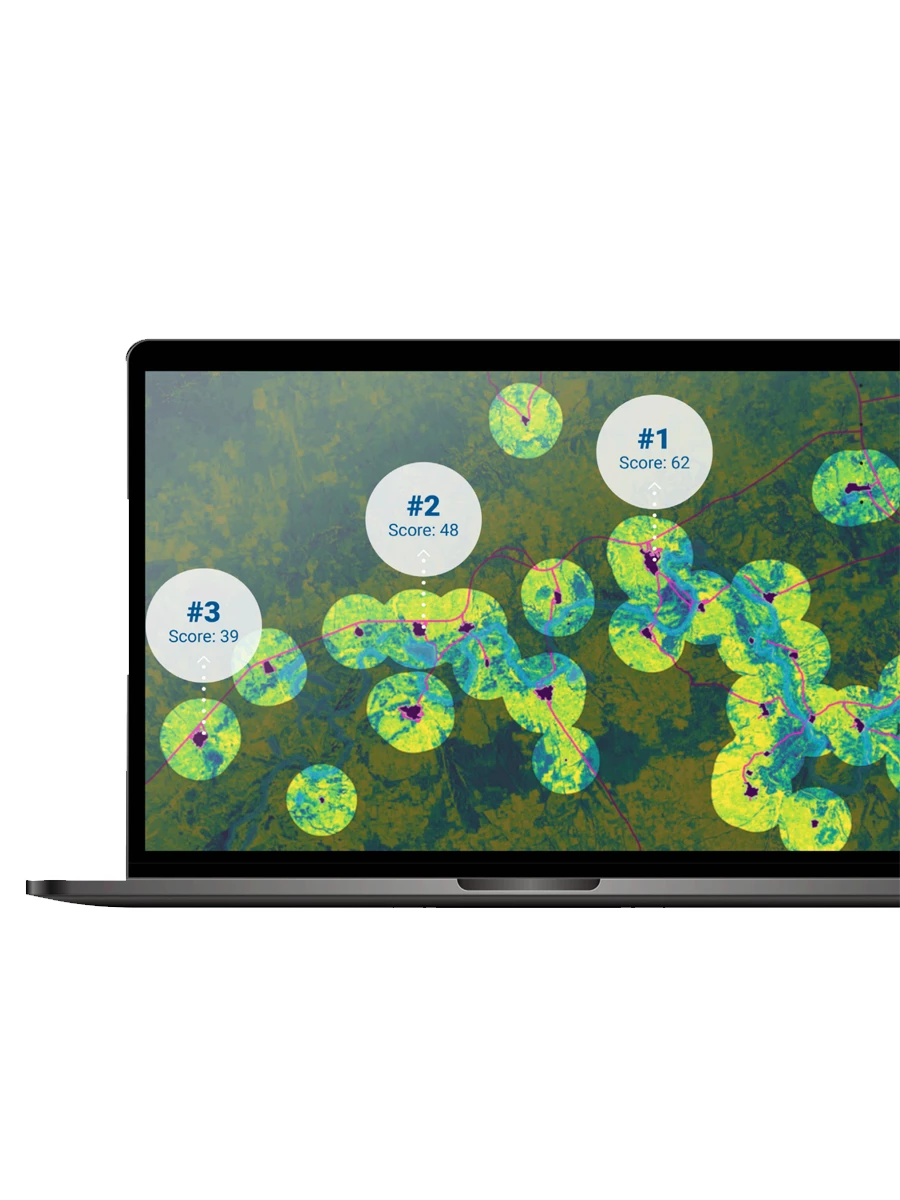
Data-poor markets inhibit investment
It is developing countries that are particularly affected by this lack of infrastructure. Development organizations, NGOs and companies have been trying to remedy this problem for years, but with moderate success. The problem is not a lack of ideas, but a lack of reliable data. Investments cannot be planned adequately, and the impact of aid projects in rural villages cannot be measured because data is incomplete, non-existent or outdated.
In stark contrast to data-saturated economies, there is not enough reliable real-time decision-making information in developing countries to make sense of investments. In data-poor markets, governments often do not know where villages are located, how many people live there, where their power grid extends, where health centers are located, and where most people need functioning health centers. They cannot plan optimal investments in rural health infrastructure based on the needs of villages. So billions are wasted without improving people's lives.
Satellites as a data source
Currently, if you want to know the location of villages, population size or coverage density, you have to travel there in person. Collecting data that can be exploited requires a great deal of effort. This is still possible on the scale of small aid projects - but no one can personally survey two billion people.
Projects like Village Data Analytics, or VIDA for short, are therefore collecting satellite data to fill the information gaps. AI-based algorithms do this by analyzing satellite imagery and on-ground data to uncover gaps in coverage, complete maps, and show the shortest routes. Planners and electrification companies can thus invest in the electrification of healthcare centers in a targeted manner.
Governments, businesses, and investors, can learn about where they can strategically and effectively target to help as many people as possible. A concrete example is the "VIDA vs. COVID" project, which identifies health centers without access to the power grid and calculates metrics such as travel time, population served, level of service and access to infrastructure. The results are displayed in a large area of interest selected by the customer in an interactive map.
Machine learning and local validation
To obtain robust data from satellite imagery, machine learning and self-optimizing algorithms are used. For example, these evaluate night light satellite imagery from NASA, and use a shortest path prediction model to test whether the health center is already connected to the national power grid. Another algorithm identifies the extent and characteristics of settlements near health facilities using daylight satellite imagery from ESA's Sentinel-2 sensor. The software extracts features such as the size and density of settlements, location and temporal characteristics of nearby water bodies, road access, and agricultural use.
Satellite images that have been taken for years or decades can be used for time series analysis. This allows the history of each settlement to be tracked and predictive modeling to be performed: How much will a village grow in the coming years? How much will its economic performance change? These questions can be modeled with the data obtained.
However, the results do not come from the algorithms' calculations alone. Surveys and on-site data collection, as well as data streams from IoT devices already deployed in existing mini-grids (smart meters), are used for validation. These data often come from governments and companies themselves. The World Bank also provides datasets needed for granular data analysis.
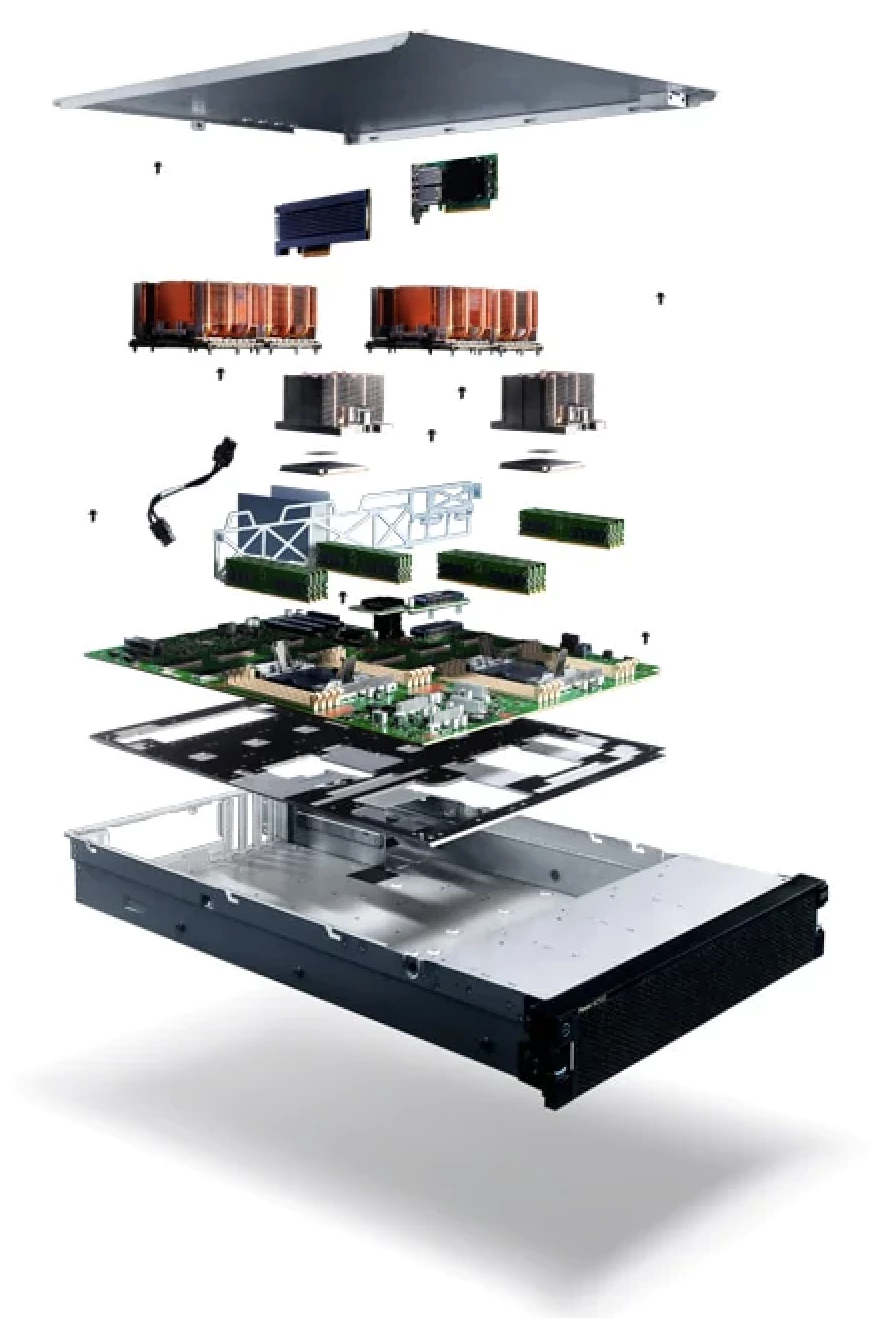
How IBM helped VIDA work with large-scale earth observation data sets
One use case, where a massive amount of data has to be processed in order to train a neural network, is the identification of water bodies from satellite imagery. The distance from a village to a water body and its seasonal changes in shape and volume are vital factors for strategic electrification decisions. There are traditional approaches to find water bodies in satellite imagery, which utilize the near infrared data the sensors provide. However, they underperform in comparison to modern machine learning solutions.
The original training data for the deep learning algorithm Deepwatermap is about 1 terabyte in size, exceeding the capabilities of most computers for (re-)training the detection model. However, the IBM® Power System™ AC922 servers and the Watson Machine Learning toolkit are perfectly equipped for handling these massive datasets and can be used to train such neural networks efficiently. The AC922 delivers unprecedented performance for analytics, artificial intelligence (AI), and modern HPC. The AC922 is able to deploy flexible models, has open frameworks and includes software tools within a contained ecosystem. On the Software side Watson Machine Learning (WML) supports the projects and provides a full range of tools and services. WML fully automate the training process for rapid prototyping to tools that gives a complete control to create a model that matches for Machine Learning models. The powerful system out of Software and Infrastructure enables VIDA to retrain the machine learning model from scratch and include newly available satellite imagery or ground truth data.
Success factors: experience and technology
The success of "VIDA vs. COVID" is to improve access to functional health care for rural populations in sub-Saharan Africa, thereby increasing the resilience of these countries to the COVID pandemic and other health risks. Currently, up to 80 percent of rural health facilities are not electrified. Because of its strategic nature, this can help hundreds of millions of people gain access to electricity and healthcare.
However, technology alone cannot deliver the success needed. A team of experts is important in any use case, but deployment in and for developing countries requires a special combination of local experience, market knowledge and technical expertise. Maintaining a team on the ground is essential: only on the ground will it be clear which parameters are important, and only on the ground can the solutions found be applied and tested.
The digital backbone
In concrete terms, such projects help to electrify entire regions, for example. This is the important and essential prerequisite for the next step: digitization. People and markets that are cut off from the power grid have no access to the globalized digital market and fall behind others.
Another important application is the optimal placement of infrastructure such as radio towers. Even when building schools or hospitals, possible locations to reach as many people as possible can be better determined. In addition, the data also provide retailers with a reliable source of information on the areas that have been insufficiently mapped so far and where they can offer products such as solar systems, water pumps or agricultural equipment.
In the information society, location is increasingly becoming a secondary factor. Here, digitization offers the opportunity to serve the global market with solutions and services - and to efficiently promote the local market with digitized services. This creates a digital backbone for areas that were previously closed off from digitization. This achievement is vital in the long term and offers a tremendous opportunity for developing countries.