Humans and AI working together typically do a better job than either could on their own. People’s innate wariness toward AI presents an impediment, but one that can be overcome by fostering fruitful cooperation, which breeds trust. Hence, the objective of this text is to suggest an alternative, pragmatic perspective on the trust issue when it comes to working with AI in a company.
When working with AI, sooner or later the topic of trust comes up.
“We don’t trust the system to make responsible decisions” or “if we can’t see exactly how the algorithm came up with all the individual steps, we can’t trust it” are typical statements describing mistrust towards artificial intelligence.
This lack of trust is particularly challenging, because AI’s biggest potential lies in humans and AI working together and complementing each other. This is most clearly on display in games, but it is no less true in the working world. Yet, something is holding us back from making full use of that potential. And that something is within us.
Since it is obvious that trust plays such an important role in this relationship, let’s think about that a little bit.
Humans trusting humans vs. humans trusting AI
At the beginning of interpersonal relations, there usually is a leap of faith, and most of our life together is based on mutual trust. To start off with a leap of faith has paid off from an evolutionary perspective and makes life much easier than mistrusting everyone from the beginning. The British journalist, businessman and writer Matt Ridley even suggests in his “The Origins of Virtue” that it has paid off to such an extent that cooperation itself might be in our DNA, as genes which generated reciprocal altruistic behavior would have been likely to be passed on. And according to psychoanalyst Erik Erikson, the development of basic trust is the first state of psychosocial development, occuring during the first two years of life.
Trust, communication, reputation and mechanisms to regulate opportunistic behavior are the foundation of successful cooperation. Game theory has demonstrated beautifully that it is actually in our own best interest to renounce short-term profit maximization when cooperating with other human beings, and to instead care for the common good. To summarize, trust is one of the key factors that lead us humans to cooperate with each other (figure 1).
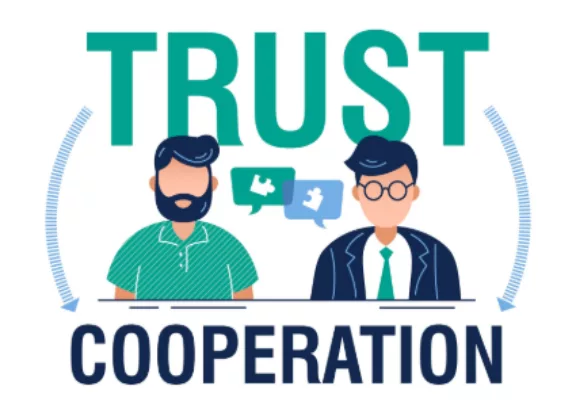
Figure 1: Trust between humans leads to cooperation
Alas, these age-old mechanisms do not transfer very well to human-AI-interaction. Perhaps if AI resembled humans more closely, we would be more inclined to extend to it the same basic trust that we do to each other. For the time being, however, we have to find ways to overcome our emotional reluctance to cooperate with AI, which we know on a rational level can be far superior to just humans or just AI working alone.
How to increase trust in AI
This begs the question of how trust in AI could be increased. Interestingly, there is research suggesting that trust is better understood as a result of, rather than a precondition for, cooperation. That is to say, if we know what part of the job an algorithm is supposed to do, we understand how it does it, and we can see it making a solid attempt at its goal, we are going to trust it. The practical implication, then, would be to make it easier to learn about how a given AI works and to integrate it into one’s workflow in an accountable manner. Trust should then be the natural consequence of successful cooperation (figure 2).
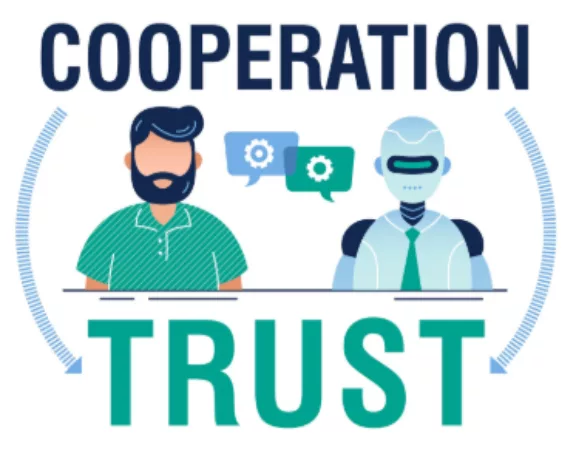
Figure 2: Cooperation between human and AI leads to trust
A lot of effort is already going into the design side of facilitating human-AI-cooperation (think human-centered design), working out systems with which humans can more easily collaborate. If we complement these efforts from the other side, and give people more opportunities to learn about AI on a broader level – especially its actual capabilities and limits – we would foster human-AI-cooperation and at the same time work on demystifying AI.
The European Commission has expanded on this line of thought in its recently published “Ethics guidelines for trustworthy AI” . According to the guidelines, trustworthy AI should be (1) lawful, respecting all applicable laws and regulations; (2) ethical, respecting ethical principles and values; and (3) robust, both technically and in terms of its social environment. The aim of the guidelines is to promote trustworthy AI as well as to set out a framework for achieving it. The third of these objectives is of particular interest to the subject at hand. When aiming to work with AI, one of the major objectives has to be making AI more reliable, explainable and thus understandable for humans.
As we try to foster tangible, traceable and transparent human-AI-interaction on a larger scale, we may look to other countries such as Finland, which has set out to teach one percent of the country’s population the basic concepts at the root of artificial technology (https://www.elementsofai.com/), thus giving people easy access to AI and the chance to familiarize with it in a conducive way.
To many employees, even though they personally might be very interested in learning more about AI in general and how they could benefit from working with it, AI still seems to be more of a curiosity than an actual thing that they can integrate into their daily work lives. One lesson to draw from the discussion above is that we need to increase the number of touch points people have with AI, such as demos and training opportunities for all employees of a company. Also, visualizations that companies put up their walls and that work as a communication tool by displaying the changes lying ahead (“change pictures”) can help decrease the perceived distance between humans and AI. Last, but not least, more actively rewarding human-AI-cooperation works as an additional motivator on the way to developing a positive AI culture in an organization.
Artificial intelligence has become a key priority for corporations. However, the more they deal with the integration of AI, the clearer it becomes which barriers they must overcome on the path to its successful integration and adoption. Based on ten in-depth interviews and discussions and interactions with dozens of companies we have identified eight barriers that need to be mastered to successfully apply AI.
Artificial intelligence (AI) as the next logical step in digitalization has become increasingly important in recent years as it enables companies to further optimize and automate their processes and develop new products and business models to make their businesses future-proof. However, the development and implementation, as well as the necessary adoption of AI systems, hold more obstacles and risks than some may expect. Resource procurement is complex and arduous: the demand for relevant skills on the job market far exceeds the supply. The data required are often not available, not accessible or not of sufficient quality. With the drive to acquire more date, data handling issues arise, along with concerns on the ethical implications of widespread adoption of data based decision systems. Governments are responding with stricter regulatory frameworks (such as the european GDPR), creating a complex and rapidly changing environment.
Based on 10 in-depth interviews with company representatives from various industries, the following eight main barriers to AI implementation and adoption were identified:
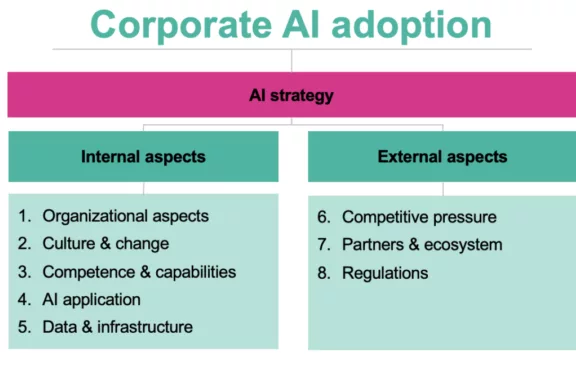
Figure 1: Overview of the main barriers to AI implementation and adoption
1. Organizational aspects
So far, many companies lack the understanding of how and where AI competencies should be anchored in their organizational structure. This is accompanied by the unclear distribution of responsibilities and tasks, such as the development of prototypes or the management of data, as well as the need for the definition of collaboration models.
2. Culture & Change
In times of uncertain and fast-moving market changes, organizations feel the pressure to open their businesses in order to be more innovative and collaborate with their ecosystem, and this also applies to the development of AI applications. Especially in large companies, cultural changes require a lot of time and strong management.
In addition to the cultural aspect, the importance of strategic change management is (still) underestimated in many companies. Instead of an integrated change support along the implementation process, isolated (communication) measures are often the only ones applied.
3. Competence & Capabilities
For many companies, the slow AI progress can be attributed to a talent shortage of relevant AI experts. All companies included in the study see significant challenges in attracting and retaining new talent. The internal upskilling of employees may be one way to reduce the problem, but it does not replace AI experts’ years of specialized education and experience.
In order to retain new hires in the long term, companies must ensure that they develop attractive and transparent career paths and meet further upcoming requirements, such as .
But even with the necessary AI resources on board, which primarily include AI experts (from the field of mathematics, statistics or equivalent studies), data scientists and engineers, and software engineers, companies face the challenge of handling interdisciplinary teams which need well-structured management due to their different backgrounds and working methods.
4. AI application
Considering the application of AI , it is known that perceived high compatibility and perceived low complexity of a system contribute to faster user adoption. This is especially true of AI systems, because lack of predictability and explainability and therefore often unintuitive results of the AI system result in a perceived higher complexity imposing a barrier to adoption. This means that AI applications must be carefully designed, as this is central to ensuring that the end user will trust and cooperate with it.
5. Data & Infrastructure
One major obstacle mentioned in every interview is the lack of quality data. The key questions arising are about what data is needed, whether it is available, how it can be obtained, who has access to it and whether its quality is sufficient.
6. Competitive pressure
From a traditional market perspective, the AI pioneers participating in our study do not have much to fear and are far ahead of their competitors. However, these days we must expand our definition of the competitive field: many technology companies are now also perceived as relevant competitors and bring AI solutions to the market, which increase expectations on the supplier and customer side putting higher pressure on companies.
7. Partners & Ecosystem
The scarcity of talent and data resources in particular raises a big question for many companies: How can we successfully implement AI under these circumstances? Many of the interviewees confirm that they use their ecosystem to drive AI progress forward. The evolving challenges at this point are the question of the right partners and the agreement on joint targets.
8. Regulations
AI developments depend, among other things, on the country in which the company operates. Stricter data protection regulations fostering a more ethical handling of data, for example, can slow down the AI progress dramatically. Whether this is an advantage or disadvantage for companies opens up a big discussion. The fact is, however, that without data no training of AI systems can be conducted.
Many companies share the same challenges in AI implementation and adoption. Therefore, it is a good option for many companies to become part of a network in order to profit from the exchange with AI experts and other companies.
This article is based on the research for the master thesis “Success factors for the implementation and adoption of applied artificial intelligence within established companies” by Laura A. Solvie (2019) that was developed in cooperation with appliedAI and the Dr. Theo Schöller – Endowed Chair for Technology and Innovation Management, TUM.